Analysis of Covariance: Nonlinear Relationship Between Covariates and Response
- Andrew Yan
- Sep 4, 2024
- 2 min read
It's often claimed that analysis of covariance (ANCOVA) is biased or invalid if a nonlinear relationship exists between covariates and the response variable. This is yet another widespread misconception regarding ANCOVA in the context of randomized clinical trials (RCTs).
Suppose the true relationship between a covariate Ⅹ and the response variable Υ is represented by a nonlinear function 𝑓(Ⅹ). Consider the following three different models:
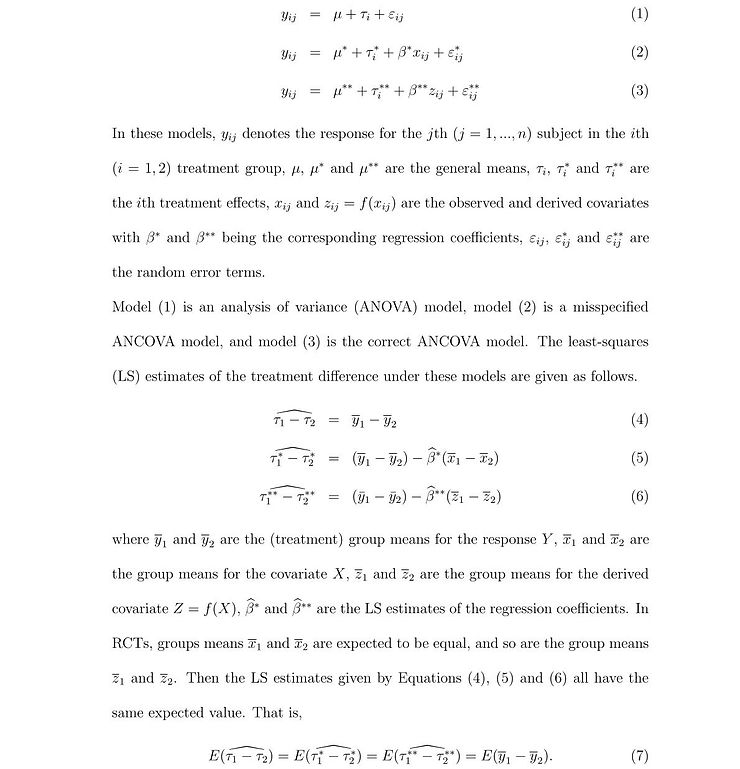
The equations in (7) indicate that both ANCOVA models (2) and (3) provide unbiased estimates of the treatment effect, provided that ANOVA model (1) is valid. However, ANCOVA model (3) is expected to be more efficient than ANCOVA model (2) because it accurately captures the true relationship between the covariate and the response variable, thereby reducing a greater portion of the error variance.
To enhance understanding, we consider a special case where 𝑓(Ⅹ) = 𝑙𝑛(Ⅹ) and evaluate the performance of the three models via simulations. The data are generated under the assumption that Υ and 𝑍 = 𝑙𝑛(Ⅹ) are bivariate normal random variables with 𝐸(Υ) = 0.5 for 𝑖 = 1, 𝐸(Υ) = 0 for 𝑖 = 2, 𝐸(𝑍) = 0, 𝑉𝑎𝑟(Υ) = 1, 𝑉𝑎𝑟(𝑍) = 1, and a correlation of 0.5. We note that model (3) represents the conditional model derived from the joint distribution of Υ and 𝑍 and is, therefore, the correct ANCOVA model. A sample size of 𝑛 = 64 per treatment group is used so that the ANOVA t-test achieves approximately 80% power. The table below shows the mean values of the estimated treatment difference (Difference) and its standard error (StdErr), along with the testing power (Power) based on 20,000 simulations.

As expected, all three models produce unbiased estimates of the treatment effect (the actual treatment difference is 0.5), and the two ANCOVA models are more efficient than the ANOVA model. Additionally, ANCOVA model (2) is less efficient than ANCOVA model (3) due to the misspecification of the covariate term. Therefore, a nonlinear relationship between covariates and the response should not be a concern for treatment group comparisons using ANCOVA in RCTs. As John Tukey aptly noted, "Adjustments are not expected to be perfect - they are only supposed to help."
Comments